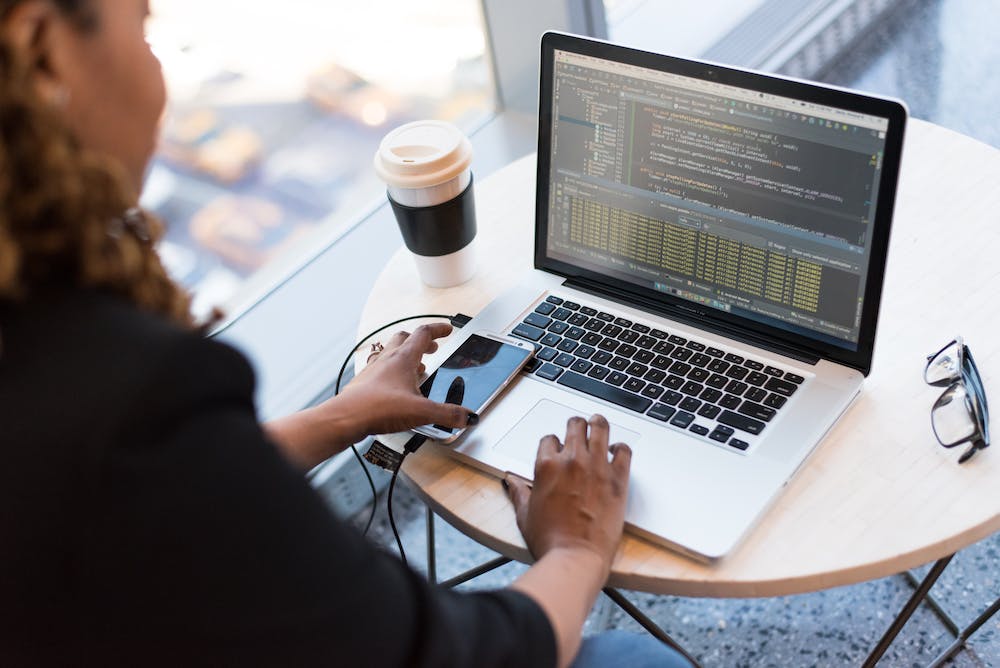
Artificial Intelligence (AI) has become one of the most transformative technologies in recent years, with advances in machine learning and deep learning leading the way. This article will explore the rise of AI, focusing on the key concepts of machine learning and deep learning, their applications, and their impact on various industries.
Understanding Machine Learning
At its core, machine learning is a subset of AI that enables machines to learn from data and make predictions or decisions without being explicitly programmed. IT involves the use of algorithms that can learn from and make predictions or decisions based on input data.
Machine learning algorithms can be broadly categorized into three types: supervised learning, unsupervised learning, and reinforcement learning. In supervised learning, the algorithm is trained on labeled data, whereas in unsupervised learning, the algorithm learns from unlabeled data. Reinforcement learning involves learning from feedback or rewards from the environment.
Applications of Machine Learning
Machine learning has a wide range of applications across various industries. In healthcare, it can be used for diagnosing diseases and predicting patient outcomes. In finance, it can help detect fraud and make investment decisions. In marketing, it can be used for customer segmentation and personalization. In manufacturing, it can optimize production processes and predictive maintenance.
Exploring Deep Learning
Deep learning is a subset of machine learning that uses neural networks with multiple layers to learn from data. These neural networks are inspired by the structure and function of the human brain, and they can automatically discover patterns and features from the data without human intervention.
One of the key advantages of deep learning is its ability to handle a large amount of unstructured data, such as images, videos, and text. This has led to significant advancements in computer vision, natural language processing, and speech recognition.
Applications of Deep Learning
Deep learning has found applications in a wide range of fields. In healthcare, it can be used for medical imaging analysis and drug discovery. In autonomous vehicles, it can enable object detection and real-time decision-making. In e-commerce, it can enhance recommendation systems and personalized shopping experiences. In cybersecurity, it can help detect and prevent cyber attacks.
The Impact of Machine Learning and Deep Learning
The rise of machine learning and deep learning has had a profound impact on various industries. These technologies have enabled organizations to derive valuable insights from data, automate repetitive tasks, and make more accurate predictions and decisions.
For example, in healthcare, machine learning algorithms can analyze medical imaging data to detect diseases at an early stage, leading to better patient outcomes. In finance, deep learning models can analyze large volumes of data to identify patterns and anomalies, helping to make more informed investment decisions.
The Future of AI: Opportunities and Challenges
As AI continues to advance, there are numerous opportunities and challenges that lie ahead. On one hand, AI has the potential to revolutionize industries, improve efficiency, and enhance human capabilities. On the other hand, there are concerns about the ethical implications of AI, including privacy, bias, and job displacement.
Additionally, the increasing complexity of AI models and the need for large amounts of data raise questions about transparency, interpretability, and accountability. As AI becomes more integrated into our daily lives, it is essential to address these challenges and ensure that AI is developed and deployed responsibly.
Conclusion
In conclusion, the rise of artificial intelligence, particularly the advancements in machine learning and deep learning, has transformed the way we approach data, make decisions, and solve complex problems. The applications of these technologies span across various domains, offering immense potential for innovation and progress. However, it is crucial to address the ethical, societal, and regulatory implications of AI to ensure that it is developed and utilized in a way that benefits society as a whole.
FAQs
What is the difference between machine learning and deep learning?
Machine learning is a broader concept that encompasses various types of algorithms that can learn from data and make predictions or decisions. Deep learning is a specific type of machine learning that uses neural networks with multiple layers to automatically discover patterns and features from data.
What are some popular tools and libraries for machine learning and deep learning?
Popular tools and libraries for machine learning and deep learning include TensorFlow, PyTorch, scikit-learn, Keras, and Theano. These tools provide a wide range of capabilities for building and deploying machine learning and deep learning models.
How can businesses leverage machine learning and deep learning?
Businesses can leverage machine learning and deep learning for a variety of applications, such as predictive analytics, recommendation systems, image and speech recognition, and process optimization. These technologies can help businesses gain a competitive edge by extracting valuable insights from data and automating complex tasks.
What are some considerations for implementing machine learning and deep learning in an organization?
When implementing machine learning and deep learning in an organization, it is important to consider factors such as data quality, model interpretability, scalability, and ethical implications. Additionally, building a team with the right expertise and ensuring proper infrastructure and governance are critical for successful implementation.
How can businesses improve their AI strategy?
Businesses can improve their AI strategy by investing in data quality and infrastructure, fostering a culture of continuous learning and experimentation, and staying informed about the latest advancements in AI. It is also important to prioritize ethical considerations and engage with stakeholders to ensure that the AI strategy aligns with the organization’s values and goals.