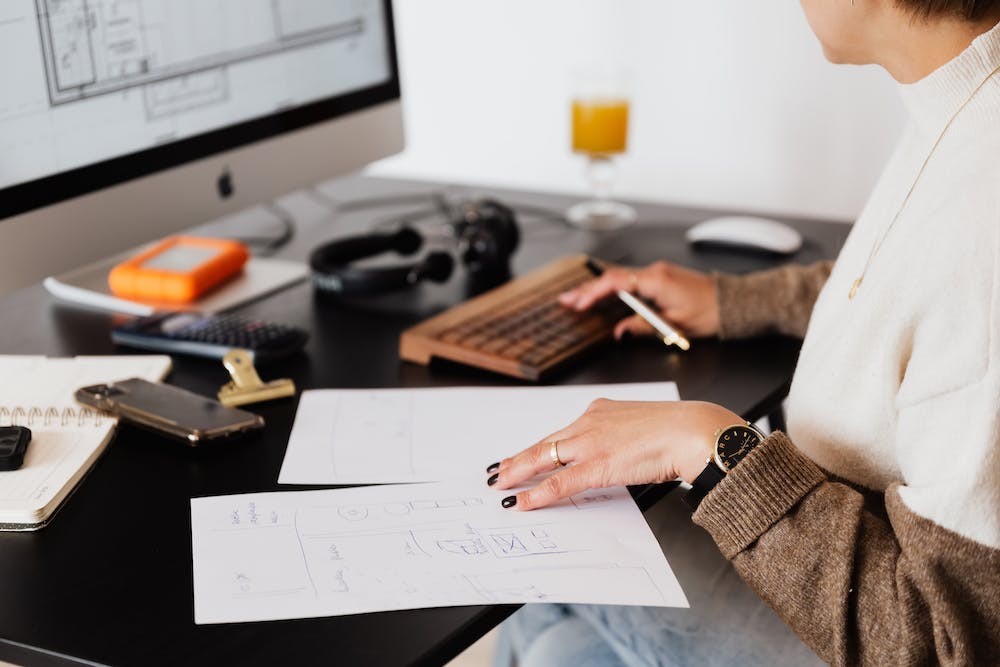
A Review of Recent Advances in Artificial Intelligence Research
Introduction
What is Artificial Intelligence?
Artificial Intelligence (AI) refers to the creation of machines and software that demonstrate human-like intelligence. This branch of computer science aims to simulate, replicate, or mimic human cognitive processes, such as learning, problem-solving, and decision-making. Recent years have witnessed significant advancements in AI research, leading to groundbreaking applications across various fields. In this article, we will delve into some of these cutting-edge developments.
Advancements in Natural Language Processing
The Rise of Transformer-based Models
One of the most notable breakthroughs in AI research is the development of transformer-based models, particularly exemplified by the introduction of the Transformer architecture in 2017. Transformer models have revolutionized natural language processing (NLP) tasks, allowing for more efficient text generation, language translation, and sentiment analysis. This innovation has led to the emergence of advanced language models such as GPT-3, which can generate coherent human-like text with minimal human input.
Transfer Learning for NLP
Another significant advancement in NLP is the widespread use of transfer learning. Transfer learning techniques involve training a model on a large dataset and then fine-tuning IT for specific tasks with a smaller dataset. This approach has greatly improved the performance of NLP models, allowing them to generalize well across various applications. By leveraging pre-trained models, developers can save substantial computational resources and accelerate the training process. The rise of transfer learning has democratized NLP, enabling faster development of practical applications.
Impressive Developments in computer Vision
Object Detection and Segmentation
Recent years have witnessed remarkable progress in computer vision, particularly in the areas of object detection and segmentation. State-of-the-art models, such as Faster R-CNN and YOLO, have displayed exceptional accuracy in detecting and localizing objects in images or videos. These models utilize anchor-based methods, which involve predicting bounding boxes and class probabilities for potential objects within an image. Such advancements have empowered applications like autonomous vehicles, facial recognition systems, and surveillance technologies.
Generative Adversarial Networks (GANs)
Generative Adversarial Networks (GANs) have also revolutionized computer vision by enabling the generation of realistic images. GANs consist of a generator network that tries to produce artificial data resembling real data while a discriminator network classifies whether the generated data is real or fake. This adversarial training process leads to progressively better image generation, with GANs able to produce photo-realistic images, impressive artwork, and even deepfake videos. GANs hold immense potential for creative industries and virtual reality applications.
Deep Reinforcement Learning
Milestone Achievements through Reinforcement Learning
Reinforcement Learning (RL) is a subfield of AI that focuses on training agents to learn from interactions with an environment. Recent advancements in deep RL have resulted in milestone achievements, mainly due to the development of algorithms like Deep Q-Networks (DQN). DQN, introduced by DeepMind, demonstrated superior performance in playing complex games, surpassing human level in Atari games and the board game Go. Such breakthroughs have not only advanced the field of gaming but also have implications for autonomous systems and robotics.
Application in Robotics
The synergy between deep RL and robotics has opened up exciting possibilities for automation. Robots trained using deep RL algorithms have shown impressive capabilities in performing complex tasks, such as object manipulation, grasping, and even agile locomotion. Deep RL allows robots to learn through trial and error, leading to more adaptable and intelligent systems. Increased deployment of robotic assistants in industries such as manufacturing, healthcare, and logistics is expected, further driving advancements in the field.
Conclusion
In conclusion, recent advances in artificial intelligence research have significantly transformed various domains, offering unprecedented opportunities for innovation and automation. Natural Language Processing has benefited from the rise of transformer-based models and transfer learning, leading to more accurate and human-like language models. computer vision applications have greatly improved with state-of-the-art object detection, segmentation, and GANs for realistic image generation. Deep reinforcement learning has achieved milestone accomplishments, impacting gaming, robotics, and autonomous systems. As AI continues to progress, IT is vital to address ethical concerns and ensure responsible development and deployment.
FAQs
What are the potential risks associated with AI?
While AI holds immense potential, there are associated risks that need to be considered. These include biases in training data leading to discriminatory decisions, job displacement due to automation, and the potential for AI systems to be hacked or manipulated. IT is crucial to develop ethical frameworks and guidelines to mitigate these risks and ensure the responsible use of AI.
What are the future prospects of AI research?
The future of AI research is promising, with ongoing efforts to enhance the capabilities and advancements in various AI subfields. The development of more powerful hardware, increased availability of large datasets, and continued innovations in algorithms are expected to further propel the field. AI is poised to revolutionize industries like healthcare, transportation, and finance, improving efficiency and decision-making processes. However, challenges related to privacy, ethics, and regulation need to be addressed for AI to reach its full potential.
How can AI benefit society?
AI has the potential to benefit society in numerous ways. IT can improve efficiency in industries, allowing for cost savings and increased productivity. AI-powered healthcare systems can enhance diagnosis and treatment accuracy. Autonomous vehicles can reduce accidents and traffic congestion. Additionally, AI can provide effective solutions for climate change, resource management, and disaster response. By leveraging AI’s capabilities, we can create a more sustainable and inclusive society.